Virtual talk
Solving the Feature Freshness Challenge in Real-Time ML
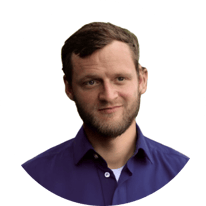
Stephan Claus
Director of Data Analytics
HomeToGo
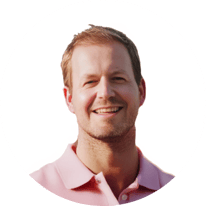
Alex Dean
CEO and co-founder
Snowplow
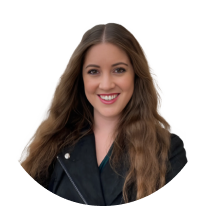
Alex Gnibus
technical evangelist
Tecton
How HomeToGo Leverages Live User Signals with Tecton and Snowplow
The difference between good and great model performance often comes down to how quickly you can capture and act on recent user signals. While batch features are a good start, incorporating live user behavior is crucial for delivering relevant results in real-time applications like recommendation engines and fraud detection. But it’s a massive engineering challenge to reduce the time between when data becomes available, and when it can be used for inference.
Join us to learn how HomeToGo, the SaaS-enabled marketplace with the world’s largest selection of vacation rentals, addressed this challenge with a real-time ML infrastructure that can efficiently process both historical patterns and live signals. Stephan Claus, Director of Data Analytics at HomeToGo, will share how the engineering team productionized live user data into fresh features by implementing Tecton and Snowplow.
In this architectural walkthrough, you’ll discover:
- Why HomeToGo evolved beyond offline features to incorporate live user behavior data
- How they implemented feature pipelines that handle multiple data velocities, ensuring models have reliable access to high-quality data for each feature type (batch, streaming and request-time)
- Technical details of their Tecton + Snowplow stack, which captures comprehensive customer behavior data, computes rich features, and serves them online at low latency
This session is ideal for engineering teams who need to go beyond batch processing to deliver better real-time results. With fresher features, ML teams can increase the accuracy and timeliness of predictions for critical, customer-facing use cases.